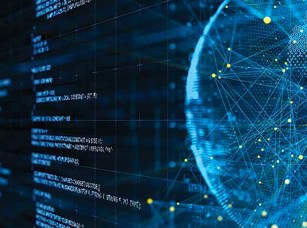
Groundbreaking new approach to generating human-driver benchmarks
Paper: Establishing a Crash Rate Benchmark Using Large-Scale naturalistic human ridehail data
Paper: Establishing a Crash Rate Benchmark Using Large-Scale naturalistic human ridehail data
A significant challenge in understanding human driving performance within an ODD is that different driving environments (e.g., limited access highways vs urban streets) result in different crash rates. Thus, it is important to compare crash rates from driving in similar environments (road type, time of day, etc.). While publicly available national crash datasets have detail on the types of locations of crashes, datasets on vehicle miles traveled do not. Moreover, national crash datasets are limited to police-reported crashes, which include only the more damaging or injurious crashes. Given these data issues, publicly available national datasets cannot produce human crash rate estimates that are appropriate to the urban ridehail driving environment.
This paper presents a study of human driving performance by ridehail drivers operating in San Francisco. The goal of the study was to generate a crash rate estimate that could be used as a human benchmark representing the crash rate for ridehail drivers driving in a low-speed and dense urban driving environment. Moreover, this environment was specifically limited to driving in the initial target San Francisco-based ODD of Cruise vehicles to further refine the relevance of the estimate.
See what Louise Zhang, VP Safety and Systems, Cruise is saying about UMTRI groundbreaking work